A Blueprint for Scalable & Reliable Enterprise AI/ML Systems // Panel // AIQCON
MLOps.community - A podcast by Demetrios
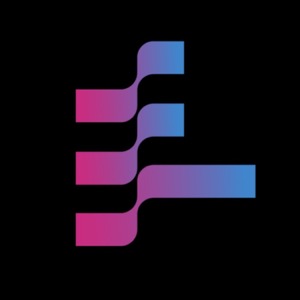
Categories:
This is a Panel taken from the recent AI Quality Conference presented by the MLOps COmmunity and Kolena // Abstract Enterprise AI leaders continue to explore the best productivity solutions that solve business problems, mitigate risks, and increase efficiency. Building reliable and secure AI/ML systems requires following industry standards, an operating framework, and best practices that can accelerate and streamline the scalable architecture that can produce expected business outcomes. This session, featuring veteran practitioners, focuses on building scalable, reliable, and quality AI and ML systems for the enterprises. // Panelists - Hira Dangol: VP, AI/ML and Automation @ Bank of America - Rama Akkiraju: VP, Enterprise AI/ML @ NVIDIA - Nitin Aggarwal: Head of AI Services @ Google - Steven Eliuk: VP, AI and Governance @ IBM A big thank you to our Premium Sponsors Google Cloud & Databricks for their generous support! Timestamps: 00:00 Panelists discuss vision and strategy in AI 05:18 Steven Eliuk, IBM expertise in data services 07:30 AI as means to improve business metrics 11:10 Key metrics in production systems: efficiency and revenue 13:50 Consistency in data standards aids data integration 17:47 Generative AI presents new data classification risks 22:47 Evaluating implications, monitoring, and validating use cases 26:41 Evaluating natural language answers for efficient production 29:10 Monitoring AI models for performance and ethics 31:14 AI metrics and user responsibility for future models 34:56 Access to data is improving, promising progress